In today’s tech-savvy world, AI isn’t just a cool add-on anymore — it’s a game-changer. You’ve probably heard about how AI is revolutionizing industries, streamlining operations, and boosting revenue. But here’s the hard truth: while many companies are experimenting with AI, only a few have figured out how to truly scale it. If you’ve been stuck in the “pilot project” phase, you’re not alone. This guide is for leaders who are ready to go beyond dabbling and actually make AI a meaningful part of their organization.
Why Scaling AI Actually Matters
Let’s be honest — launching a pilot AI project feels like a win. It’s exciting, innovative, and makes you look like you’re ahead of the curve. But pilots aren’t the finish line. Without scaling, those AI wins remain isolated — like having one smart employee while the rest of the team’s in the dark.
Why Should You Care About Scaling?
- You get real business value — not just demos
- You improve consistency across operations
- You stay competitive while others fall behind
Look at Amazon. Their AI didn’t just stay in product recommendations — it now powers logistics, Alexa, warehouses, and beyond.
Crafting a Vision That Everyone Believes In
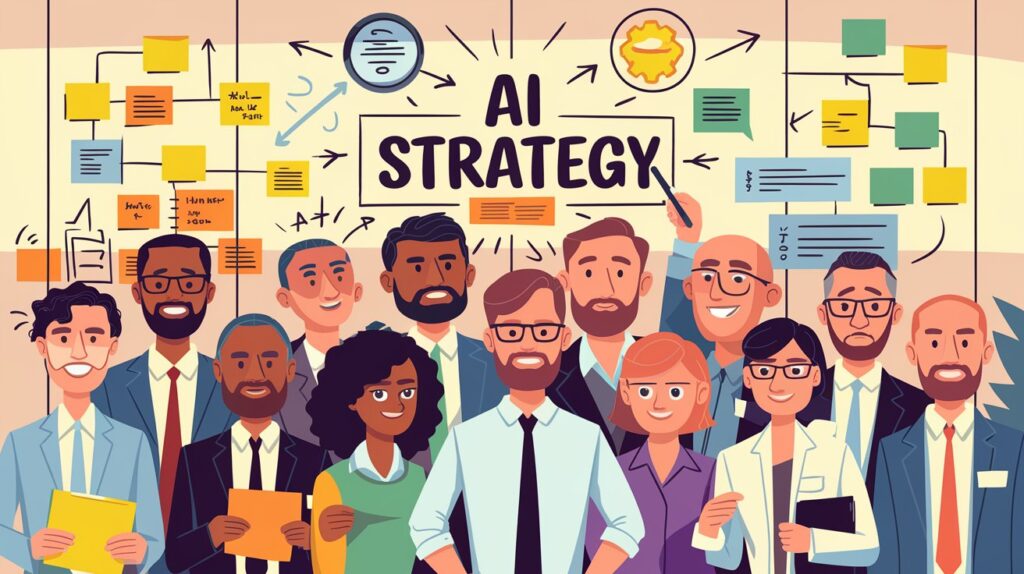
Before you start pouring money into tools and talent, take a moment to ask: What’s our AI north star? Are you trying to boost sales? Cut costs? Deliver faster customer service?
Tips to Build a Smart AI Strategy:
- Tie your AI goals to clear business outcomes
- Choose a few use cases that are doable but impactful
- Map out a timeline — think short wins and long-term plans
And yes — leadership buy-in is huge. If your C-suite isn’t on board, your AI journey might fizzle before it even gets rolling. Get your top people excited. Show them the numbers. Better yet, show them real examples of what competitors are doing.
Get Your Data House in Order
Imagine trying to cook a five-star meal with rotten ingredients. That’s what building AI on bad data is like. Scaling AI starts with trustworthy, well-organized data.
Here’s what to focus on:
- Accessibility: Teams need easy access to the right data
- Quality: Clean, structured, and labeled data matters more than volume
- Infrastructure: Set up systems that grow with you — whether that’s cloud, on-prem, or hybrid
Real Talk: Most companies underestimate how messy their data is. Don’t make that mistake. Invest early in good data governance. It saves time and sanity later.
Assemble a Crew That Can Actually Do the Work
AI isn’t magic — it’s built by people. And to scale it, you need a crew that knows their stuff.
Key Roles to Bring Onboard:
- Data Scientists who can build models
- Engineers who can deploy and scale
- Product Managers who can connect tech to business value
- Domain Experts who understand your company inside out
Upskill or Hire? Both. Upskilling builds loyalty and keeps tribal knowledge intact. Hiring brings in fresh perspective. You might also consider strategic partnerships with AI vendors or academic institutions.
Tip from the Field: Create internal “AI evangelists” — enthusiastic employees who act as the bridge between departments and help smooth out communication.
Picking Tools That Don’t Turn into Headaches
With a zillion AI tools out there, it’s easy to fall into analysis paralysis. Start with your needs, not the hype.
Essential Tools & Tech:
- Cloud Platforms: AWS SageMaker, Azure ML, Google Vertex AI
- ML Libraries: TensorFlow, PyTorch, scikit-learn
- MLOps Tools: MLflow, Kubeflow, Weights & Biases
Look for:
- Easy deployment and scaling
- Built-in monitoring and feedback loops
- Compatibility with your existing systems
Pro Insight: Don’t get lured into buying the most expensive or most popular tool. Choose what fits your team’s skillset and business goals.
Be Ethical, Be Compliant, Be Responsible
AI isn’t just about cool outputs — it’s about responsible choices. Scaling AI means facing real-world questions about bias, fairness, and privacy.
Key Governance Moves:
- Create clear AI usage guidelines
- Appoint a team to oversee ethics and compliance
- Monitor your models regularly for bias and performance issues
Whether you’re dealing with healthcare data or consumer behavior, being transparent and fair isn’t optional. It’s a must.
Win Over the Humans (a.k.a. Change Management)
Even the best AI system fails if your people don’t use it. That’s where organizational culture comes in.
Tips to Build a Supportive Culture:
- Help employees understand that AI is here to assist, not replace
- Offer training sessions tailored to different skill levels
- Celebrate early wins and make them visible across teams
Personal Take: I’ve seen AI fail just because teams weren’t properly trained or felt threatened. It’s not just about the tech — it’s about trust.
Start Small, Scale Smart
You don’t need to roll out AI across your company overnight. Start with one or two use cases that are easy to measure and have a clear ROI.
Top Use Cases to Explore:
- Chatbots for 24/7 support
- Sales forecasting with predictive analytics
- Fraud detection in financial systems
- Document automation in HR or legal
Success Formula:
- Test small (Pilot)
- Optimize fast (MVP)
- Scale what works (Enterprise rollout)
Document everything. Use the lessons learned to improve your next project.
Keep Improving — Always
Scaling AI is not a “one-and-done” task. It’s a cycle of improvement. You must monitor, retrain, and optimize your models.
What You Should Be Doing:
- Monitor for model drift (when data patterns change)
- Set up regular retraining schedules
- Use A/B testing to evaluate performance
- Create dashboards with KPIs and insights
AI isn’t static. The more it learns, the better it gets — as long as you feed it the right data and keep tabs on it.
Expect Roadblocks — Then Bulldoze Through Them
Scaling AI isn’t all smooth sailing. But knowing the bumps ahead makes them easier to navigate.
Challenge | Smart Fix |
---|---|
Siloed data | Break down barriers with centralized platforms |
Skills shortage | Invest in training and strategic hiring |
Privacy concerns | Build governance frameworks from day one |
Cost pressures | Use scalable cloud solutions and open-source tools |
Cultural resistance | Lead with transparency and empathy |
Stay Ready for What’s Next
AI moves fast. What’s hot today might be outdated tomorrow.
To Stay Ahead:
- Explore LLMs (like ChatGPT or Claude) for internal knowledge support
- Consider Edge AI for real-time decision-making
- Stay curious — keep attending conferences, reading, and experimenting
Remember: Scaling AI isn’t just about today. It’s about building a system that evolves with your business.
Wrapping Up
Scaling AI isn’t easy, but it’s worth it. With a clear vision, the right team, a healthy data ecosystem, and continuous learning, your organization can go from AI curiosity to AI powerhouse.
Take it one step at a time. Focus on outcomes. And don’t forget — it’s a journey, not a race.
FAQs
Q: How long does it take to scale AI?
A: Usually 12–36 months depending on goals, industry, and readiness.
Q: Can smaller businesses scale AI?
A: Absolutely. Tools today are affordable and scalable, even for SMEs.
Q: What are the biggest hurdles?
A: Siloed data, lack of skilled talent, unclear goals, and weak leadership support.